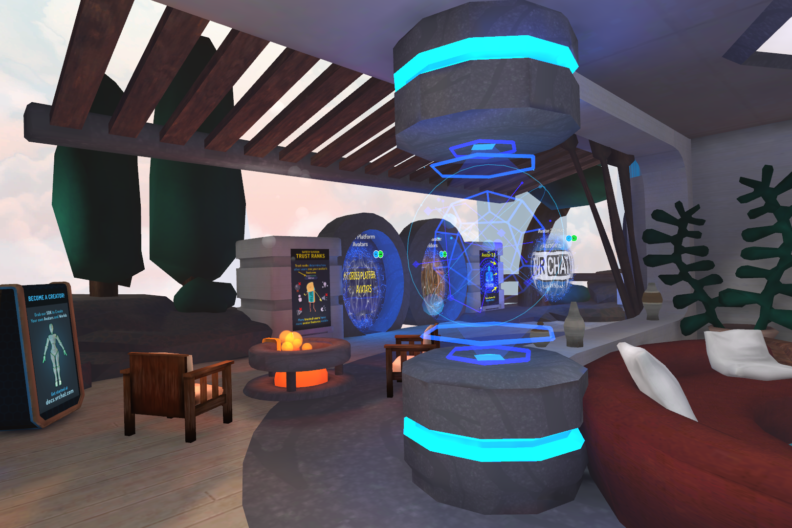
VIViD Seminars
Welcome to VIViD seminars. To sign up for the seminar mailing list please email [email protected]
If you are interested to become a speaker, please contact: [email protected]
Here are the seminars that have already been done:
Seasonal Monitoring of Rivers and Lakes at Global Scales with Deep Learning Methods
Patrice Carbonneau
3rd MAY 2023
I began my university studies with bachelor’s degrees in both physics (Université de Sherbrooke, Sherbrooke, Canada) and engineering (Université Laval, Québec, Canada). These degrees gave me the technical skills and the mathematical background that have done much to shape my contributions to physical geography. I eventually came to fluvial geomorphology through the study of turbulence and sediment transport during my master’s degree (INRS-ETE, Québec, Canada). The study of a complex problem such as turbulence prompted an interest in complex phenomena in rivers and thus I undertook a Ph.D. (INRS-ETE, Québec, Canada) on the intergranular void spaces that constitute the habitat of juvenile atlantic salmon. In addition to gaining an understanding of salmonid habitat, the requirements of my Ph.D. brought me to develop an expertise in the field of remote sensing applied to fluvial environments. Namely, during a Ph.D. internship as a visiting scholar in Fitzwilliam College, Cambridge, I developed skills in digital photogrammetry which were completed with a second internship at the School of geography of the University of Leeds. My post-doctoral work, carried out jointly at the INRS-ETE in Quebec, the School of Geography at the University of Leeds and the department of geography of Durham university, built on my knowledge of remote sensing and salmonid habitat to develop pioneering methods for the catchment-scale characterization of salmonid habitat with high resolution airborne remote sensing methods.
This interest in high-resolution imagery has led me to research topographic mapping from Unmanned Aerial Vehicles (UAV or drones) and since 2008 I have conducted sucessfull UAV field campaigns in the UK, the Swiss Alps, the Atacama Desert and Svalbard. Further, recent work has built upon knowledge of fluvial remote sensing, drone imagery and ecology and moved to a larger scale problem: the health and condition of the Ganges. My objectve over the next decade is to make a data
Virtual Material Creation in a Material World
8 March 2023
Valentin Deschaintre
In the last 20 years, virtual 3D environments have become an integral parts of many industries. Of course, the video game and cinema industries are the most prominent examples of this, but cultural heritage, architecture, design, medicine, all benefit from virtual environment rendering technologies in different ways. These renderings are computed by simulating light propagation in a scene with various geometries and materials. In this talk, I will focus on how materials define the light behaviour at the intersection with a surface, and most importantly how they are created nowadays. I will then discuss recent research and progress on material authoring and representation, before concluding with open challenges to further help artists reach their goals faster.
Valentin is a Research Scientist at Adobe Research in the London lab, working on virtual material creation and editing. He previously worked in the Realistic Graphics and Imaging group of Imperial College London hosted by Abhijeet Ghosh. He obtained his PhD from Inria, in the GraphDeco research group, under the supervision of Adrien Bousseau and George Drettakis. During his PhD he spent 2 months under the supervision of Frédo Durand, at MIT CSAIL. His Thesis received the French Computer Graphics Thesis award 2020 and UCA Academic Excellence Thesis Award 2020. His research cover material and shape (appearance) acquisition, creation, editing and representation, leveraging deep learning methods.
Do You Speak Sketch?
1 March 2023
Yulia Gryaditskaya
Sketches are the easiest way to externalize and accurately convey our vision and thoughts. In my talk, I will discuss our ability to create and understand sketches, how this task can be accomplished by a machine, as well as how AI can help humans to become more fluent in sketching. I will discuss differences and similarities in working with single-object sketches and scene sketches. Continuing our journey of understanding freehand sketches, one might observe that sketches are a two-dimensional abstraction of a three-dimensional world. Studying how to generate a sketch from a 3D model is an old problem, yet not fully solved. In my talk, I will focus on the inverse problems of retrieving and generating 3D models from sketch inputs.
Dr. Yulia Gryaditskaya is Assistant Professor in Artificial Intelligence at CVSSP and Surrey Institute for People-Centred AI, UK, and she is a co-director of the SketchX group. Her primary research interests lie in AI, sketching, and CAD for creation and creativity. Before joining CVSSP, she was a postdoctoral researcher (2017-2020) at Inria, GraphDeco, under the guidance of Dr. Adrien Bousseau, where she studied concept sketching techniques and worked on sketch-based modeling. She received her Ph.D. (2012-2016) from MPI Informatic, Germany, advised by Prof. Karol Myszkowski and Prof. Hans-Peter Seidel. Her Ph.D. focused on High Dynamic Range (HDR) image calibration, capturing HDR video on a mobile device, tone mapping of HDR content, structured light fields, and editing materials in such light fields. While working on my Ph.D., she spent half a year in the Color and HDR group in Technicolor R&D, Rennes, France, under the guidance of Dr. Erik Reinhard. She received a degree (2007-2012) in Applied Mathematics and Computer Science with a specialization in Operation Research and System Analysis from Lomonosov Moscow State University, Russia.
Some Research Problems in Spanish Art (And How Computer Science Techniques Could Potentially Solve Them)
22 February 2023
Andrew Beresford
This seminar will advance a discussion of some current problems in Spanish art research with a view to understanding how collaborative research synergies in Computer Science could potentially address them. The first part of the discussion will be based on the relationship between physical artefacts and the spaces in which they are displayed, making specific reference to the impact agenda, while the second will focus on particular problems of representation, discussing how the human body functions as a semiotic signifying system, conveying a range of meanings to the observer.
Andrew M. Beresford is Professor of Medieval and Renaissance Studies at the University of Durham, and has published widely on Iberian art and literature, focusing principally on the cults of the saints and the signifying potential of the human body. His most recent book (2020) offered a study of the flaying of St Bartholomew.
Geographical Applications of High-resolution Data
15 February 2023
Rebecca Hodge
My research uses high-resolution data to address questions about how water and sediment move in river channels. My aim in this seminar is to introduce you to the questions and techniques that we are interested in, but also to identify potential areas where this work might benefit from computer science expertise. I will cover a range of different projects, including: 1) work where we recorded and analysed the sound made by rivers to predict the depth of water in the river; 2) an project using terrestrial laser scanner 3D point data to quantify the topographic properties of river beds, which is part of a larger project aiming to link the shape of the river bed to the flow resistance (i.e. how much the bed slows down the water); and 3) a project using 3D XCT scan data to measure the arrangement of individual sediment grains in a gravel river bed, with the aim of working out the forces required to entrain those grains from the bed.
Professor Rebecca Hodge is a Professor in the Department of Geography, Durham University. Her research interests are primarily in the area of fluvial geomorphology, with a particular focus on sediment transport. She uses a combination of numerical modelling and field measurements to quantify sediment and flow processes. In both approaches she is interested in the application of new techniques, for example the use of Cellular Automaton models, CT scanning and Terrestrial Laser Scanning, and 3D printing. A key focus of her research is the way in which small scale processes upscale, for example the way in which the properties and interactions of sediment grains affect sediment flux at the reach scale.
Geometry Guided Image Based Rendering and Editing
8 February 2023
Julien Philip
We acquire more images than ever before. While smartphones can now take beautiful pictures and videos, we often capture content under constrained conditions. For instance, lighting and viewpoint are notably hard to control. In this talk, we will discuss how we can leverage geometry from either single or multi-view inputs to enable novel view synthesis and relighting. The key idea in the work we will discuss is that providing a good representation of geometric information to neural networks allows to tackle these complex synthesis and edition tasks. We will first discuss view-synthesis and neural rendering, introducing both mesh-based and point-based neural rendering methods that exploit either rasterization, point-splatting or volumetric rendering along learnt components and discuss the key advantages and limitations of each approach. We will then discuss relighting, starting with multi-view inputs and showing how traditional computer graphics buffers can be combined with a neural network to produce realistic-looking relit images for both outdoor and indoor. We will then see how the ideas developed for multi-view can be transferred to the single view case using a neural network to do image-space ray-casting.
Julien Philip is a research scientist at Adobe Research London, before that he received his PhD in 2020 from inria Sophia Antipolis where he worked under the supervision of George Drettakis. He interned at Adobe in 2019 working with Michaël Gharbi. Before inria, Julien completed his undergraduate studies in France, with a joint degree from Télécom Paris with a major in Computer Science and ENS Paris-Saclay with a major in Applied Mathematics. His research interests are at the crossroad of Computer Graphics, Vision, and Deep Learning. During his PhD, he focused on providing editability in the context of multi-view datasets with a focus on relighting. Since then, his research is focused on neural rendering and image relighting.
Applications of Graph Convolutional Networks (GCN)
25 January 2023
Tae-Kyun Kim
Graph convolution networks have been a vital tool for a variety of tasks in computer vision. Beyond predefined array data, graph representation with vertexes and edges capture inherent data structure better. 3D shapes in mesh are probably the best example in graphs, and a set of multi-dimensional data vectors and their relations are often cast as nodes and edges in a graph. I will present two example studies of our own on GCN, published at CVPR2021. In the first work, we focus on deep 3D morphable models that directly apply deep learning on 3D mesh data with a hierarchical structure to capture information at multiple scales. While great efforts have been made to design the convolution operator, how to best aggregate vertex features across hierarchical levels deserves further attention. In contrast to resorting to mesh decimation, we propose an attention based module to learn mapping matrices for better feature aggregation across hierarchical levels. Our proposed module for both mesh downsampling and upsampling achieves state-of-the art results on a variety of 3D shape datasets. In the second work, we propose a novel pool-based Active Learning framework constructed on a sequential Graph Convolution Network (GCN). Each images feature from a pool of data represents a node in the graph and the edges encode their similarities. With a small number of randomly sampled images as seed labelled examples, we learn the parameters of the graph to distinguish labelled vs unlabelled nodes. To this end, we utilise the graph node embeddings and their confidence scores and adapt sampling techniques such as CoreSet and uncertainty-based methods to query the nodes. Our method outperforms several competitive AL baselines such as VAAL, Learning Loss, CoreSet and attains the new state of-the-art performance on multiple applications.
Tae-Kyun (T-K) Kim is an Associate Professor and the director of Computer Vision and Learning Lab at KAIST (School of Computing) since 2020, and is a visiting reader at Imperial College London, UK. He has led Computer Vision and Learning Lab at ICL, since 2010. He obtained his PhD from Univ. of Cambridge in 2008 and Junior Research Fellowship (governing body) of Sidney Sussex College, Univ. of Cambridge during 2007-2010. His BSc and MSc are from KAIST. His research interests primarily lie in machine (deep) learning for 3D computer vision, including: articulated 3D hand pose estimation, face analysis and recognition by image sets and videos, 6D object pose estimation, active robot vision, activity recognition, object detection/tracking, which lead to novel active and interactive visual sensing. He has co-authored over 80 academic papers in top-tier conferences and journals in the field, and has co-organised series of HANDS workshops and 6D Object Pose workshops (in conjunction with CVPR/ICCV/ECCV). He was the general chair of BMVC17 in London, and is Associate Editor of Pattern Recognition Journal, Image and Vision Computing Journal, and IET Computer Vision. He regularly serves as an Area Chair for the major computer vision conferences. He received KUKA best service robotics paper award at ICRA 2014, and 2016 best paper award by the ASCE Journal of Computing in Civil Engineering, and the best paper finalist at CVPR 2020, and his co-authored algorithm for face image retrieval is an international standard of MPEG-7 ISO/IEC. https://sites.google.com/view/tkkim/
Studying Human Movement through Video Recordings: Research on Indian Music Performance
11 January 2023
Martin Clayton
Human movement is an important window on musical performance in a number of ways. Manual gestures, for instance, can provide a lot of information about singing performance: how melody is conceived, how rhythm and metre are marked, and how nonverbal communication is used between performers to manage performances. Periodic aspects of movement, including body sway and repeated hand movements, can reveal rhythmic structures that are difficult to interpret from sound alone, and can also index coordination and leadership patterns between performers.
In this presentation I will give an overview of research into human movement in Indian music performance, including both qualitative and computational approaches to the analysis of video recordings. In the latter case, I will describe ongoing work to extract movement information using pose estimation, and to analyse the extracted data.
Martin Clayton is Professor in Ethnomusicology in Durham University. His research interests include Hindustani (North Indian) classical music, rhythmic analysis, musical entrainment and embodiment. His book Time in Indian Music (OUP 2000) is the main reference work on rhythm and metre in North Indian classical music, and his publications on musical entrainment have been similarly influential. He has led or collaborated on numerous interdisciplinary projects, including ‘Interpersonal Entrainment in Music Performance’ (AHRC) and ‘EnTimeMent’ (EU FET).
Retinal vasculometric analysis
30 November 2022
Sarah Barman
Type 2 diabetes is an increasing public health problem, affecting 1 in 10 adults globally, and is a major cause of premature death. Early detection and prevention both of Type 2 diabetes and associated cardiovascular disease is key to improving the health of a population. Identifying those people at high risk of disease could be improved by incorporating information from retinal fundus image analysis of the microvasculature. Growing evidence in the field links morphological features of retinal vessels to early physiological markers of disease. Recent advances in artificial intelligence applied to imaging problems can be used to advance healthcare in terms of generating efficiencies from automated assessment of images and also by providing opportunities for prediction of disease. Machine learning approaches to enable precise automated measurements of vessel shape and size have been explored with respect to arteriole/venule detection and image quality assessment.
Sarah Barman is Professor in Computer Vision in the Faculty of Engineering, Computing and the Environment at Kingston University where she leads research into retinal image analysis in the School of Computer Science and Mathematics. After completing her PhD in optical physics at King’s College London she took up a postdoctoral position for the next four years, also at King’s College, in image analysis. In 2000 she joined Kingston University. Professor Barman is a member of the UK Biobank Eye and Vision consortium, a Fellow of the Institute of Physics and is a registered Chartered Physicist. Current projects include those funded by the National Institute for Health and Care Research (NIHR) and Wellcome Trust. Previous project funding has included support from UK research councils, the Leverhulme Trust and the Royal Society. She is the author of over 100 international scientific publications and book chapters. Professor Barman’s main area of interest in research is in the field of artificial intelligence applied in the context of medical image analysis. Her work is currently focused on exploring machine learning and computer vision algorithms to solve recognition, quantification and prediction challenges.
Interesting challenges in image analysis for applications including astronomy, free-space optical communications and satellite surveillance
23 November 2022
James Osborn
When light passes through the Earth’s atmosphere it becomes distorted, causing stars to appear to twinkle. My work involves modelling, measuring and mitigating atmospheric turbulence for applications such as astronomy, free-space optical communications and satellite surveillance. These distortions limit the precision of astronomical observations, the achievable bandwidth and link availability for free-space optical communications and the precision of orbital parameters for space surveillance. In all of these applications, information is extracted from image data. It is obvious that we have not yet optimised this process, presenting an opportunity for more sophisticated image analysis techniques to make a real world impact. I will present an overview of the work from the free-space optics group in physics and discuss a few areas for potential collaboration.
I am currently an Associate Professor (Research) and UKRI Future Leaders Fellow in the Centre for Advanced Instrumentation, University of Durham, UK. Previous to this I worked as a postdoctoral fellow for two years at the Universidad Catolica, Chile. My research is concerned with forecasting, modelling, measuring and mitigating the Earth’s atmospheric turbulence for applications such as astronomy and free-space optical communications. This has led to several novel instrumentation packages deployed at some of the world’s premier observatories and to many strong international collaborations.
Digital mobility outcomes beyond the laboratory: advantages and challenges
16 November 2022
Silvia Del-Din
Digital mobility outcomes (e.g. gait) are emerging as a powerful tool to detect early disease and monitor progression across a number of conditions. Typically, quantitative gait assessment has been limited to specialised laboratory facilities. However, measuring gait in home and real-world/community settings may provide a more accurate reflection of gait performance as it allows walking activity to be captured over time in habitual contexts.
Modern accelerometer-based wearable technology allows objective measurement of digital mobility outcomes, comprising metrics of real-world walking activity/behaviour as well as discrete gait characteristics.
Quantification of digital mobility outcomes in real-world/unsupervised environments presents considerable challenges due to sensor limitations, lack of standardised protocols, definitions and outcomes, engineering challenges, and contextual recognition.
However, results from our work are encouraging regarding the use of digital mobility outcomes for application in large multi-centre clinical trials, for supporting diagnosis and guiding clinical decision making.
This presentation will address the feasibility, advantages and challenges of measuring digital mobility outcomes during real-world activity for discriminating pathology and detecting early risk. The use of traditional digital gait outcomes and novel metrics as a measurement tool for characterising patient populations, discriminating disease (e.g. Parkinson’s disease) and detecting risk (e.g. prodromal stage of disease) will also be discussed.
Dr Silvia Del Din
Newcastle University Academic Track (NUAcT) Fellow
Translational and Clinical Research Institute,
Newcastle University
I am a bioengineer by background, I completed my Bachelors’ in Information Engineering, my Master’s in Biomedical Engineering and my PhD in Information Engineering at the University of Padua. I am currently a Newcastle University Academic Track (NUacT) Fellow in the Faculty of Medical Sciences. I am part of the Brain and Movement (BAM) Research Group at the Translational and Clinical Research Institute of Newcastle University where, over the years, I have been contributing to build the digital health theme. Within the BAM group, I lead the wearable/digital technology team where I supervise the work of Research Associates and PhD students. Within the University, I am also the Early Career Researcher (ECR) representative for the Innovation, Methodology and Application Theme. This year I have been elected Chair of the Early Career Researchers Working Group of the BioMedEng association Council. My research aims at innovating digital healthcare and translating it into clinical practice. In particular, I am interested in enhancing the use of wearable technology together with innovative data analysis techniques to enable remote monitoring and clinical management in ageing and neurodegenerative disorders (e.g. Parkinson’s disease (PD)). My current research aims to provide a proof-of-concept of this vision in PD by understanding and predicting the impact that medication has on people with PD’s everyday life motor performance. This could revolutionise at-home patient care by optimising medication regimes. This is a short video that describes my research vision: https://www.youtube.com/watch?v=CpUpVp1myhg
I am an IEEE, ISPGR, IET and BioMedEng Association member. I have published >75 full papers (h-index 28). I have attracted >£800K in funding: I am PI and Co-I of various digital health related projects (e.g. MRC Confidence in Concept, IMI-EU Mobilise-D, IDEA-FAST). I am an advocate for Early Career Researchers (ECR) and I strive to be a role-model of Women in STEM.
Email: [email protected]
ORCID: ORCID-0000-0003-1154-4751
Website: https://www.ncl.ac.uk/medical-sciences/people/profile/silviadel-din.html
DP4DS: Data Provenance for Data Science
Paolo Misser
2nd Nov 2022
Data-driven science requires complex data engineering pipelines to clean and transform data in preparation for machine learning. Our aim is to provide data scientists with facilities to gain a better understanding of how each step in the pipeline affects the data, from the raw input to “learning-ready” datasets (eg training sets), both for debugging purposes and to help engender trust in the resulting models. To achieve this, we have proposed a provenance management framework and technology for generating, storing, and querying very granular accounts of data transformations, at the level of individual elements within datasets whenever possible. In this talk, we present the essential elements of the proposal, from formal definitions, to a specification of a flexible and generic provenance capture model targeted to python / Pandas programs, and with an overview of the architecture we designed in response to scalability challenges. The plan is to conclude the talk with a demo of our current prototype (given recently at VLDB’22), and with an open discussion on how this work may impact data science working practices.
Paolo Misser is currently a Professor of Scalable Data Analytics with the School of Computing at Newcastle University and a Fellow (2018-2022) of the Alan Turing Institute, UK’s National Institute for Data Science and Artificial Intelligence. After first and MSc degrees in Computing at Universita’ di Udine, Italy (1990), and a further MSc in Computer Science from University of Houston (USA), my career began as Research Scientist at Bell Communications Research, USA (1994-2001), working on Software and Data Engineering for next-generation telco services. After a 3-year spell as independent consultant for the Italian Government and as adjunct professor at Universita’ Milano Bicocca, in 2004 I moved on to University of Manchester, School of Computer Science where in 2018 I completed my PhD in Computer Science, and where I stayed on as postdoc until 2011, worklng in Prof. Carole Goble’s eScience group. I then moved to Newcastle in 2011 as a Lecturer, progressing directly to Reader and then, in 2020, Professor. At Newcastle I lead the School of Computing’s post-graduate academic teaching on Big Data Analytics, as well as a UG module on Predictive Analytics. In our School, I co-lead the Scalable Computing Group. I am Sr. Associate Editor for the ACM Journal on Data and Information Quality (JDIQ).
Towards Explainable Abnormal Infant Movements Identification for the Early Prediction of Cerebral Palsy
Edmond Ho
26th Jul 2022
Providing an early diagnosis of cerebral palsy (CP) is key to enhancing the developmental outcomes for those affected. Diagnostic tools such as the General Movements Assessment (GMA), have produced promising results in early prediction, however, these manual methods can be laborious. In this talk, I will introduce the projects we have been working on closely with our clinical partners since 2018. We focused on using the pose-based features extracted from skeletal motions for abnormal infant movement detection using traditional machine learning as well as deep learning approaches. We further enhanced the interpretability of the proposed models with intuitive visualization.
Edmond Shu-lim Ho is currently an Associate Professor and the Programme Leader for BSc (Hons) Computer Science in the Department of Computer and Information Sciences at Northumbria University, Newcastle, UK. Prior to joining Northumbria University in 2016 as a Senior Lecturer, he was a Research Assistant Professor in the Department of Computer Science at Hong Kong Baptist University. He received his PhD degree from the University of Edinburgh. His research interests include Computer Graphics, Computer Vision, Motion Analysis, and Machine Learning.
The Universal Vulnerability of Human Action Recognition (HAR) Classifiers and Potential Solutions
He Wang
23th Mar 2022
Deep learning has been regarded as the `go to’ solution for many tasks today, but its intrinsic vulnerability to malicious attacks has become a major concern. The vulnerability is affected by a variety of factors including models, tasks, data, and attackers. In this talk, we investigate skeleton-based Human Activity Recognition (HAR), which is an important type of time-series data widely used for self-driving cars, security and safety, etc. Very recently, we have identified a universal vulnerability in existing HAR classifiers. This is through proposing the first adversarial attack approaches on such tasks. Further, we also investigate how to enhance the robustness and resilience of existing classifiers, across different data, tasks, classifiers and attackers.
He Wang is an Associate Professor in the Visualisation and Computer Graphics group, at the School of Computing, University of Leeds, UK. He is also a Turing Fellow, an Academic Advisor at the Commonwealth Scholarship Council, the Director of High-Performance Graphics and Game Engineering, and an academic lead of Centre for Immersive Technology at Leeds. His current research interest is mainly in computer graphics, vision and machine learning and applications. Previously he was a Senior Research Associate at Disney Research Los Angeles. He received his PhD and did a post-doc in the School of Informatics, University of Edinburgh.
Deep Learning for Healthcare
Xianghua Xie
16th March 2022
In this talk, I would like to discuss some of our recent attempts in developing predictive models for analysing electronic health records and understanding anatomical structures from medical images. In the first part of the talk, I will present two studies of using electronic health records to predict dementia patient hospitalisation risks and the onset of sepsis in an ICU environment. Two different types of neural network ensemble are used, but both aim to provide some degrees of interpretability. For example in the dementia study, the GP records of each patient were selected one year before diagnosis up to hospital admission. 52.5 million individual records of 59,298 patients were used. 30,178 were admitted to hospital and 29,120 remained with GP care. From the 54,649 initial event codes, the ten most important signals identified for admission were two diagnostic events (nightmares, essential hypertension), five medication events (betahistine dihydrochloride, ibuprofen gel, simvastatin, influenza vaccine, calcium carbonate and colecalciferol chewable tablets), and three procedural events (third party encounter, social group 3, blood glucose raised). They performed significantly above conventional methods. In the second part of the talk, I would like to present our work on graph deep learning and how this can be used to perform segmentation on volumetric medical scans. I will present a graph-based convolutional neural network, which simultaneously learns spatially related local and global features on a graph representation from multi-resolution volumetric data. The Graph-CNN models are then used for the purpose of efficient marginal space learning. Unlike conventional convolutional neural network operators, the graph-based CNN operators allow spatially related features to be learned on the non-Cartesian domain of the multi-resolution space. Some challenges in graph deep learning will be briefly discussed as well.
Xianghua Xie is a Professor at the Department of Computer Science, Swansea University. His research covers various aspects of computer vision and pattern recognition. He started his lecturing career as an RCUK academic fellow, and has been an investigator on several projects funded by EPSRC, Leverhulme, NISCHR, and WORD. He has been working in the areas of Pattern Recognition and Machine Intelligence and their applications to real world problems since his PhD work at Bristol University. His recent work includes detecting abnormal patterns in complex visual and medical data, assisted diagnosis using automated image analysis, fully automated volumetric image segmentation, registration, and motion analysis, machine understanding of human action, efficient deep learning, and deep learning on irregular domains. He has published over 170 research papers and (co-)edited several conference proceedings.
Video Understanding – An Egocentric Perspective
Dima Damen
23rd Feb 2022
This talk aims to argue for a fine(r)-grained perspective onto human-object interactions, from video sequences, captured in an egocentric perspective (i.e. first-person footage). Using multi-modal input, I will present approaches for determining skill or expertise from video sequences [CVPR 2019], few-shot learning [CVPR2021], dual-domain [CVPR 2020] as well as multi-modal fusion using vision, audio and language [CVPR 2021, CVPR 2020, ICCV 2019, ICASSP 2021]. These approaches centre around the problems of recognition and cross-modal retrieval. All project details at: https://dimadamen.github.io/index.html#Projects
I will also introduce the latest on EPIC-KITCHENS-100, the largest egocentric dataset in people’s homes. [http://epic-kitchens.github.io] and the ongoing collaboration Ego4D [https://ego4d-data.org/]
Dima Damen is a Professor of Computer Vision at the University of Bristol. Dima is currently an EPSRC Fellow (2020-2025), focusing her research interests in the automatic understanding of object interactions, actions and activities using wearable visual (and depth) sensors. She has contributed to novel research questions including assessing action completion, skill/expertise determination from video sequences, discovering task-relevant objects, dual-domain and dual-time learning as well as multi-modal fusion using vision, audio and language. She is the project lead for EPIC-KITCHENS, the largest dataset in egocentric vision, with accompanying open challenges. She also leads the EPIC annual workshop series alongside major conferences (CVPR/ICCV/ECCV). Dima is a program chair for ICCV 2021, associate editor of IJCV, IEEE TPAMI and Pattern Recognition. She was selected as a Nokia Research collaborator in 2016, and as an Outstanding Reviewer in CVPR2021, CVPR2020, ICCV2017, CVPR2013 and CVPR2012. Dima received her PhD from the University of Leeds (2009), joined the University of Bristol as a Postdoctoral Researcher (2010-2012), Assistant Professor (2013-2018), Associate Professor (2018-2021) and was appointed as chair in August 2021. She supervises 9 PhD students, and 5 postdoctoral researchers.
Vision != Photo
Yi-Zhe Song
9th Feb 2022
While the vision community is accustomed to reasoning with photos, one does need to be reminded that photos are mere raw pixels with no semantics. Recent research has recognised this very fact and started to delve into human sketches instead — a form of visual data that had been inherently subjected to human semantic interpretation. This shift has already started to cause profound impact on many facets of research on computer vision, computer graphics, machine learning, and artificial intelligence at large. Sketch has not only been used as novel means for applications such as cross-modal image retrieval, 3D modelling, forensics, but also as key enablers for the fundamental understanding of visual abstraction and creativity which were otherwise infeasible with photos. This talk will summarise some of these trends, mainly using examples from research performed at SketchX. We will start with conventional sketch topics such as recognition, synthesis, to the more recent exciting developments on abstraction modelling and human creativity. We will then talk about how sketch research has redefined some of the more conventional vision topics such as (i) fine-grained visual analysis, (ii) 3D vision (AR/VR), and (iii) OCR. We will finish by highlighting a few open research challenges to drive future sketch research.
Yi-Zhe Song is a Professor of Computer Vision and Machine Learning, and Director of SketchX Lab at the Centre for Vision Speech and Signal Processing (CVSSP), University of Surrey. He obtained a PhD in 2008 on Computer Vision and Machine Learning from the University of Bath, a MSc (with Best Dissertation Award) in 2004 from the University of Cambridge, and a Bachelor’s degree (First Class Honours) in 2003 from the University of Bath. He is an Associate Editor of the IEEE Transactions on Pattern Analysis and Machine Intelligence (TPAMI), and Frontiers in Computer Science – Computer Vision. He served as a Program Chair for the British Machine Vision Conference (BMVC) 2021, and regularly serves as Area Chair (AC) for flagship computer vision and machine learning conferences, most recently at CVPR’22 and ICCV’21. He is a Senior Member of IEEE, a Fellow of the Higher Education Academy, as well as full member of the EPSRC review college.
Meta-Learning for Computer Vision and Beyond
Timothy Hosepedales
2th Feb 2022
In this talk I will first give an introduction to the meta-learning field, as motivated by our recent survey paper on meta-learning in neural networks. I hope that this will be informative for newcomers, as well as reveal some interesting connections and contrasts that will be thought-provoking for experts. I will then give a brief overview of recent meta-learning applications which shows how it can be applied to benefit broad issues in computer vision and beyond and including dealing with domain-shift, data augmentation, learning with label noise, improving generalisation, accelerating reinforcement learning, and enabling Neuro-Symbolic learning.
Timothy Hospedales is a Professor within IPAB in the School of Informatics at the University of Edinburgh, where he heads the Machine Intelligence Research group. He is also the Principal Scientist at Samsung AI Research Centre, Cambridge and a Turing Fellow of the Alan Turing Institute. His research focuses on data-efficient and robust machine learning using techniques such as meta-learning and lifelong transfer-learning, in both probabilistic and deep learning contexts. He works in a variety of application areas including computer vision, vision and language, reinforcement learning for robot control, finance and beyond.
Known Operator Learning – An Approach to Unite Machine Learning, Signal Processing and Physics
Andreas Maier
27th Oct 2021
We describe an approach for incorporating prior knowledge into machine learning algorithms. We aim at applications in physics and signal processing in which we know that certain operations must be embedded into the algorithm. Any operation that allows computation of a gradient or sub-gradient towards its inputs is suited for our framework. We derive a maximal error bound for deep nets that demonstrates that inclusion of prior knowledge results in its reduction. Furthermore, we show experimentally that known operators reduce the number of free parameters. We apply this approach to various tasks ranging from computed tomography image reconstruction over vessel segmentation to the derivation of previously unknown imaging algorithms. As such, the concept is widely applicable for many researchers in physics, imaging and signal processing. We assume that our analysis will support further investigation of known operators in other fields of physics, imaging and signal processing.
Prof. Andreas Maier was born on 26th of November 1980 in Erlangen. He studied Computer Science, graduated in 2005, and received his PhD in 2009. From 2005 to 2009 he was working at the Pattern Recognition Lab at the Computer Science Department of the University of Erlangen-Nuremberg. His major research subject was medical signal processing in speech data. In this period, he developed the first online speech intelligibility assessment tool – PEAKS – that has been used to analyze over 4.000 patient and control subjects so far.
From 2009 to 2010, he started working on flat-panel C-arm CT as post-doctoral fellow at the Radiological Sciences Laboratory in the Department of Radiology at the Stanford University. From 2011 to 2012 he joined Siemens Healthcare as innovation project manager and was responsible for reconstruction topics in the Angiography and X-ray business unit.
In 2012, he returned the University of Erlangen-Nuremberg as head of the Medical Reconstruction Group at the Pattern Recognition lab. In 2015 he became professor and head of the Pattern Recognition Lab. Since 2016, he is member of the steering committee of the European Time Machine Consortium. In 2018, he was awarded an ERC Synergy Grant “4D nanoscope”. Current research interests focuses on medical imaging, image and audio processing, digital humanities, and interpretable machine learning and the use of known operators.
Visual Relevance in New Sensors, Robots and Skill Assessment
Walterio Mayol-Cuevas
1st Dec 2021 [4pm]
In this talk I will cover recent and ongoing work at my group in Bristol University that looks at different aspects of relevance in visual perception. The field of Active Vision in the early days of Computer Vision already posed important questions about what should be sensed and how. In recent years these fundamental concerns are re-emerging as we want artificial systems to cope with the complexity and amount of information in natural tasks. With this motivation, I will present recent and ongoing work that looks at different aspects of how to sense and process the world from the point of view of novel visual sensors and their algorithms, robots interacting in close loop with users and computer vision methods for skill determination aimed at Augmented Reality guidance.
Walterio Mayol-Cuevas is full professor at the Computer Science Department University of Bristol in the UK and Principal Research Scientist at Amazon US. He received the B.Sc. degree from the National University of Mexico and the Ph.D. degree from the University of Oxford. His research with students and collaborators proposed some of the earliest versions of applications of visual simultaneous localization and mapping (SLAM) for robotics and augmented reality. And more recently, working on visual understanding for skill in video, new human-robot interaction metaphors and Computer Vision for Pixel Processor Arrays. He was General Co-Chair of BMVC 2013 and the General Chair of the IEEE ISMAR 2016. Topic editor of an upcoming Frontiers in Robotics and AI title for environmental mapping.
Object Recognition and Style Transfer: Two Sides of the Same Coin
Peter Hall
6th Oct 2021
Object recognition numbers among the most important problems in computer vision. State of the art is able to identify thousands of different kinds object classes, locating individual instances to pixel level in photographs with a reliability close to human.
Style transfer refers to a family of methods that makes artwork from an input photograph, in the style of an input style exemplar. Thus a portrait photograph may be re-rendered in the style of van Gough. It is an increasingly popular approach to manufacturing artwork in the creative sector.
But all object recognition systems suffer a drop in performance of up to 30% when artwork is used as input; things that are clearly visible to humans in artwork manage to evade detection. Style transfer fails to transfers all but the most trivial aspects of style – it is not just Cubism that cannot be emulated, the van Gough styles would convince nobody if presented as a forgery.
I will argue that these problems are related: that to make art any agent must understand the world visually, and that to understand the visual world robustly requires an abstract into a semantic form ripe for art making. Our prior work backs up these claims will be outlined, and the lessons learned will be put to use to advance both style transfer and object recognition, as well as support new applications in making photographic content available to people with visual impairments.
Peter Hall has been researching in the intersection of computer vision and computer graphics for more than twenty years. During that time he has developed algorithms for automatically emulating a wide range of a artwork given input photographs, including Cubism, Futurism, cave art, child art, Byzantine style art, and others. He has developed algorithms for robust object recognition, and was among the first to the interest in the “cross depiction problem”, which is recognition regardless of rendering style. Recent work includes learning artistic warps (eg Dali’s melting watches) and processing photographs into tactile art for people with visual impairment to have some access to content. Peter is professor of Visual Computer at the University of Bath, and director of a DTC: the Centre for Digital Entertainment. He has actively promoted the intersection of vision and graphics via networks, conference series, seminars etc. He has authored around 150 papers, co-authoring with many international colleagues including Art Historians.
Image Saliency Detection: From Convolutional Neural Network to Capsule Network
Jungong Han
24th Nov 2021
Human beings possess the innate ability to identify the most attractive regions or objects in an image. Salient object detection aims to imitate this ability by automatically identifying and segmenting the most attractive objects in an image. In this talk, I will share with you two recent works that we published in the top venues. In the first work, we showcase a guidance strategy for multi-level contextual information integration under the CNNs framework, while in the second work, we demonstrate how we carry out the saliency detection task using new Capsule Networks.
Jungong Han is a professor and the director of research of Computer Science at Aberystwyth University, UK. He also holds an Honorary Professorship at the University of Warwick. Han’s research interests span various topics of computer vision and video analytics, including object detection, tracking and recognition, human behavior analysis, and video semantic analysis. With his research students, he has published over 70 IEEE/ACM Transactions papers, and 19 conference papers from CVPR/ICCV/ECCV, NeurIPS, and ICML. His work has been well-received earning over 7900 citations and his H-index is 45 in Google scholar. He is the Associate Editor-in-Chief of Elsevier Neurocomputing, and the Associate Editor of several Computer Vision journals.